Why do machines fail? It’s a fundamental question that has fascinated Guillermo Morales throughout a career that has taken him halfway around the world, to the highest levels of technical research and down into the minutiae of microscopic imperfections.
A Mexican/French national now based at SKF’s Research and Technology Development Centre in the Netherlands, Morales divides his time between overseeing industrial R&D projects and holding senior academic positions at the University of Lyon (INSA) and Imperial College London. He has made numerous contributions to the science of rotating machinery. One of the most significant has been his work on new ways to predict the operating life of rolling bearings under real-world conditions.
“You can design many mechanical components by just calculating stresses and making sure you do not exceed the fatigue limit,” Morales says. “In this way you do not have to worry about fatigue. It will not happen. [But] in bearings, the duty cycles are so long, and the stresses are so high, that you almost always get fatigue. You need a mathematical formulation to understand these stresses and their effect on component life.”
The role of fatigue in bearing life is something engineers have known for a long time, but the development of models that could account for that fatigue has taken generations. “You can’t predict bearing life in a deterministic way,” Morales says. “Very small variations in operating conditions or material or geometry can have a big effect on bearing life. So you have to combine statistics with physics to make useful predictions.”
The first models that took this combined approach to bearing life prediction were published in the mid-20th century, with SKF scientists at the forefront of their development. “Those early papers introduced basic concepts that we still use today,” says Morales. “Things like the difference between the static and dynamic capacity of a bearing.”
Over the following decades, advances in theoretical understanding and practical experience paved the way for more sophisticated models. In the 1980s, for example, engineers figured out how to account for the fatigue limit in predicting bearing life – a stress level below which very little fatigue accumulates in the material.
Scratching the surface
As modelling techniques improved, so did bearings. “All these 20th century models were based mainly on subsurface fatigue,” Morales explains. “But thanks to advances in manufacturing technology, such as the introduction of very clean steels, the subsurface fatigue challenge has been largely solved.”
These modern bearings could still experience fatigue, but failures were much more likely to be triggered by a different set of causes, including poor lubrication, contamination, frictional heat or electrical damage. “In most of these modern situations, the problems are not in the body of the bearing,” Morales says. “They come from some kind of problem on the surface.”
And the surface was something Guillermo Morales knew a lot about. After earning bachelor’s and master’s degrees in mechanical engineering in his native Mexico, he travelled to the University of Cambridge in the UK to pursue a PhD in tribology.
“My PhD work was trying to model the effect and behaviour of roughness in lubricated contacts,” he says. “In tribology, ‘roughness’ is a general way of describing any microgeometric feature. It can be a scratch, an indentation or a texture on the surface.” Such surface patterns are a headache for tribologists because they disrupt the thin films of lubricant that allow mechanical components to move smoothly over long periods of time.
But roughness is difficult to model. “People used to do numerical work to model roughness,” explains Morales, “but it’s a very hard, time-consuming problem for a computer. You need a system of five equations with five unknowns distributed in time and space.”
The key to Morales’ dissertation was to find a simpler, faster way to tackle the complex mathematics of roughness. He did so by breaking it down into sinusoidal “waves”. This dramatically simplified the calculations required, while still allowing any type of surface imperfection to be modelled as a collection of different waves.
The new approach reduced the computing power required to analyse complex surfaces by several orders of magnitude compared to older methods. His time at Cambridge also gave Morales a lifelong fascination with tribology – a fascination that eventually led him to SKF.
Morales joined the company’s research laboratory in January 2000 and soon found new applications for his modelling approach. One was simulating bearing performance in mixed lubrication environments, where contamination or lack of lubricant creates areas of direct metal-to-metal contact within a bearing. Another was a new modelling approach to evaluate the effect on life of the small indentations that can occur if a bearing is mishandled during manufacturing, shipping or assembly.
Life in general
Fast forward a few years, Morales and his colleagues were successfully applying mixed lubrication and surface damage models to a wide range of problems both within SKF and with its customers. In 2012, a new technical director approached Morales with a bigger challenge. Morales recalls: “He said our bearing life models were useful, but they were too rigid. It took too much effort to adapt the model to a different problem or to integrate new knowledge.”
The technical director’s request was simple, but daunting. Could Morales and his team take what they had learned about the effect of surface conditions on bearing life and build a general-purpose model that would better predict bearing life in the real world?
Their answer to this challenge was two years in the making. “We already had some of the key ingredients,” says Morales. “To build a general-purpose bearing life prediction model, you have to simulate the operation of different bearings under different conditions over millions of cycles. Without a fast solver, that would have been impossible.”
Other parts of the model required the team to break new ground. In particular, Morales says, they had to develop an approach that combined their new surface-damage models with traditional methods for estimating subsurface fatigue.
One model, many solutions
The first iteration of the SKF Generalized Bearing Life Model (GBLM) for conventional steel bearings was introduced as a concept to customers at the 2015 Hannover Messe. It offered the promise of an immediate solution to many challenges faced daily by design engineers. “With a better life-prediction model, you can design better machines,” says Morales. “Our model helps designers select the optimal size and type of bearing for their application and allows companies to provide more reliable advice on maintenance and replacement intervals.” The result is more efficient use of resources, with fewer breakdowns and instances of premature replacement of parts that still have life left in them.
In the past decade, Morales and his colleagues have expanded the GBLM to include new types of bearings, notably adding models for the hybrid bearings now used in demanding applications ranging from turbomachinery to electric vehicle transmissions. They have also updated the approach to reflect ongoing improvements in conventional bearing technology, including new steels and better heat treatment techniques.
GBLM is also helping SKF respond to other major industry trends. For example, as companies look for ways to further reduce their consumption of resources, users are increasingly turning to remanufacturing to extend the life of large bearings. The GBLM helps these users make informed decisions about remanufacturing intervals based on the likely rate at which surface damage will accumulate in their applications.
Is there more to come? As principal scientist, Guillermo Morales now has much more on his plate than bearing life models, but he maintains a strong interest in the development of the GBLM. “We have developed a flexible and extensible way to model different bearings, operating conditions and failure modes,” he says, “but all such models need to be validated with data from experiments and tests. Advanced sensors are now giving us better insight into the conditions inside our bearings, and these insights will help us extend and improve our modelling approach.
Can you solve this math problem?
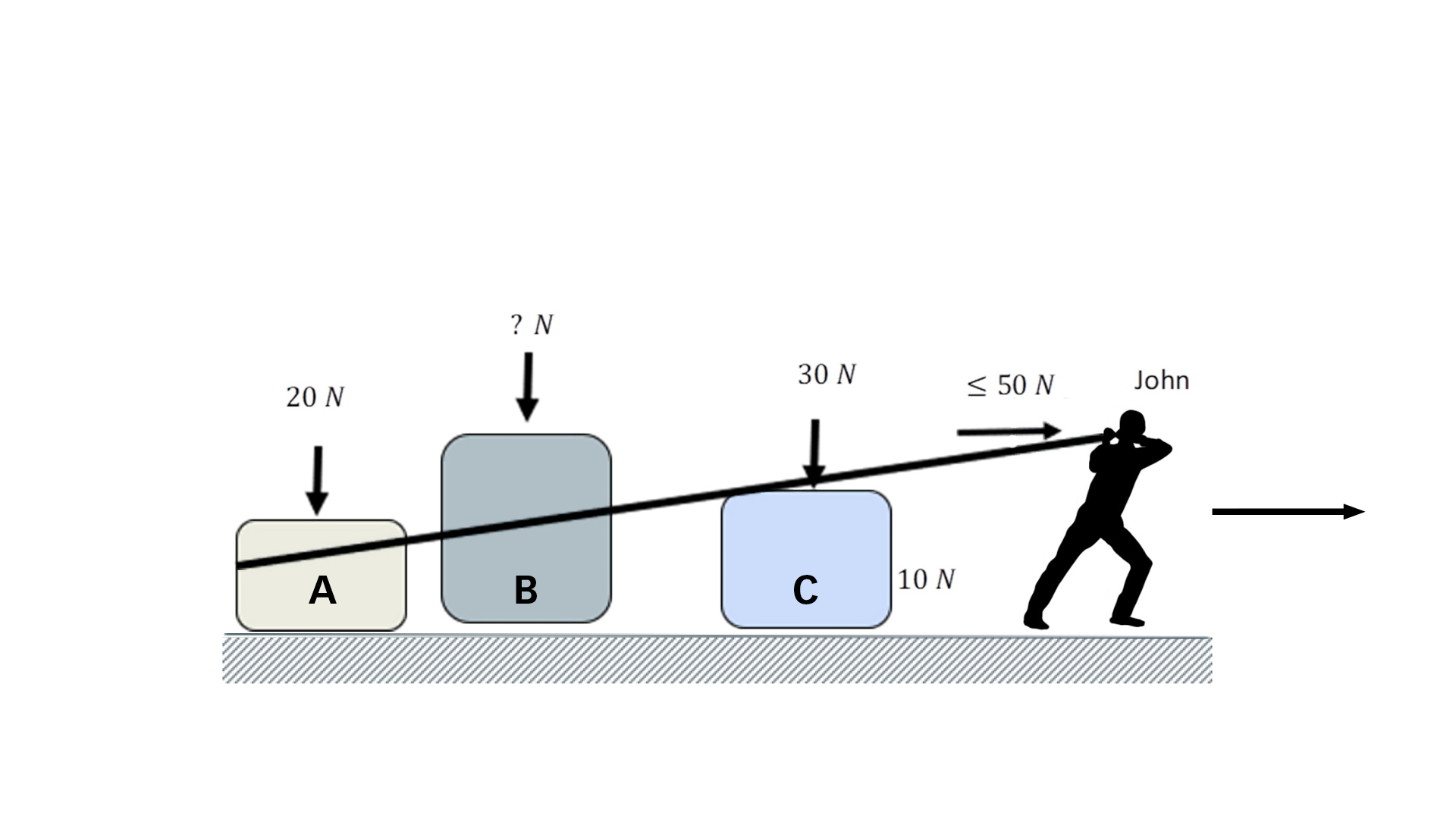
John is pulling initially block A, when it touches block B then he pulls A+B and finally A+B+C. At this moment he is exercising a horizontal force of 50N. Block A weights 20 N and block C, 30 N. It is also known that block C contributes with a force of 10 N to the 50N pulled by John. The materials and surfaces of block A and B are exactly the same, but the weight of block B is unknown. It is also known that the material and surfaces of blocks A and B produce twice the friction for equal weight of material and surface of C.
What is the weight of block B?
Background:
Amontons’ first law:
The force of friction is directly proportional to the applied load.
Amontons’ second law:
The force of friction is independent of the apparent area of contact.
Third law:
Friction force also depends on the nature of the surfaces in contact.
The post From theory to practice first appeared on Evolution.